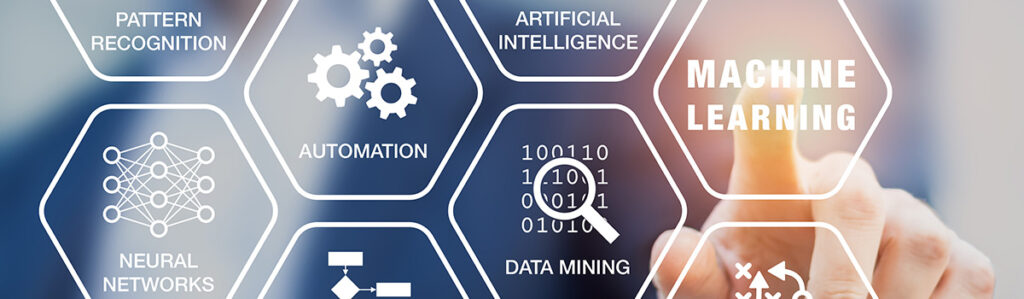
Using Machine Learning to Predict Hospitalizations
Bronx RHIO recently completed the first year of a two-year Machine Learning project. In this project, Bronx RHIO applied machine learning techniques on its data warehouse to build models to predict costly acute care utilization: preventable emergency department (ED) and inpatient (IP) encounters.
The regressing ED utilization model used over 770,000 patients’ information in the Bronx RHIO system to predict how many ED visits a patient would have based on their demographic and clinical data during the past 12 months. The model found prior ED utilization (number of visits and days between visits) and diagnosis history (substance-related disorders, nausea and vomiting, and respiratory disease)to be strong predictors of frequent ED visits.
The 30-day unplanned hospitalization readmission model, a classification model,used over 82,000 encounters to predict if there would be a readmission within 30 days after an inpatient admission based on the patient’s data during the past 13 months. Utilization of IP/ED/outpatient visits, age, and diagnosis history (fluid and electrolyte disorders, heart disease, respiratory disease, and hypertension) were among the most predictive features.
In the second year of this project, Bronx RHIO is building these predictive models into the Virtual Health Record for use by Bronx RHIO members. This feature will allow members to identify their relatively high-risk patients for ED utilization or readmission based on the predictive results. Meanwhile, new data elements and techniques are being explored and evaluated for further inclusion in the models, including lab results, vital signs (blood pressure, BMI), and assessment values (PHQ-2/9, smoking status)and social determinates of health data, such as geospatial data on socioeconomic status and homelessness.
Risk predictions will also be incorporated into BronxWorks’ “Facesheet,”a new-client summary of clinical/demographic/social data that recently went live in the Bronx RHIO. The incorporation of risk predictions will allow BronxWorks, a community-based organization specializing in housing and other social services, to better understand the clinical risk factors of their clients. Bronx RHIO looks forward to assessing how such predictions will improve BronxWorks’ ability to manage care for their clients as well as identifying other community-based organizations that may benefit from similar services.